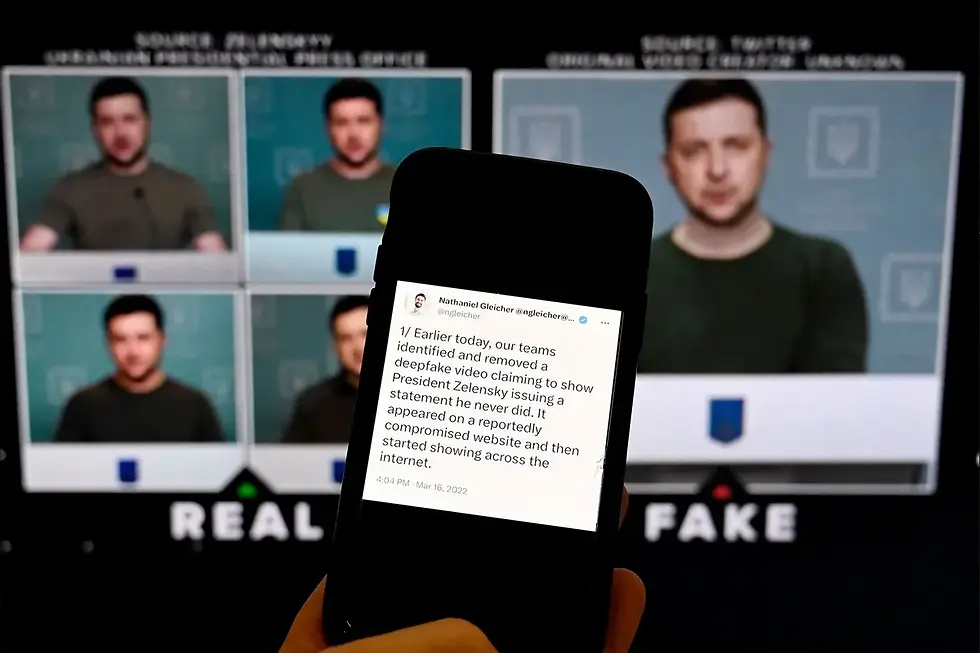
This post, co-authored by TRUE's Principal Investigator Yvonne and Advisory Board member Raquel Vázquez Llorente, was first published on Just Security on 17 June 2024. It forms part of a symposium on AI and Human Rights, edited by Jess Peake of UCLA School of Law.
Over the past 18 months, the commercialization of applications driven by artificial intelligence (AI) has revolutionized our ability to fabricate realistic images and videos of events that never occurred. Generative AI tools such as Dall-E or Midjourney help us create multiple depictions of the same event by varying the angles of image shots, making it more challenging to discern if a composition of images is real or fake. Previously, producing such content would have required the resources, computational power, and expertise that was mostly reserved for Hollywood studios. Now, building an alternative reality is accessible to many of us, for as little as $20 a month.
We are in an information environment where increasingly AI and non-AI media not only coexist but intermingle, creating a hybrid media ecosystem. Techniques like “in-painting” and “out-painting” allow users to easily add or remove objects in human-captured content without noticeable manipulation. The newest audio capabilities produce realistic outputsfrom text or a few minutes of speech that can even fool presumably sophisticated banking security systems – when combined with other types of media, this could mean that the visual content of a video is real, but the accompanying audio could be fake, altering our understanding of the event, or affecting our opinions and worldview.
Having spent much of the last decade advocating and writing about the promises and pitfalls of user-generated evidence for justice and accountability for international crimes, we see how advances in audiovisual AI are exacerbating existing challenges in the verification and authentication of content, while also presenting new complexities. In this article, we share high-level findings from our work with WITNESS and the TRUE project, exploring how synthetic media impacts trust in the information ecosystem. We also offer recommendations to address issues related to the integrity of user-generated evidence and its use in court amid the rise of AI.
Synthetic Media and User-Generated Evidence
The commercialization, ease of use, and accessibility of generative AI tools have led to an increase in the circulation of synthetic media – image, audio, or video that has been artificially produced or manipulated, especially through use of AI. This type of content is sometimes referred to as “deepfakes”, although the latter is rather a sub-category of synthetic media in which human likeness is convincingly replaced to portray an individual as saying or doing something they did not say or do.
User-generated evidence – open or closed source information generated by a person through their personal digital devices, and which may be used in legal proceedings – is increasingly being leveraged to inform judgments on atrocity crimes before both international and domestic courts. Earlier this year, it was referenced in the hearings at the International Court of Justice for the case of South Africa v. Israel. Beyond legal proceedings, user-generated media is also central to the work of United Nations human rights investigations.
At the same time, stories about images, videos, or audio fabricated using AI appear in the media almost daily. Recent examples from elections around the world, non-consensual sexual imagery, and disinformation campaigns highlight how much the term “deepfake” is now a feature of the media landscape.
What might more widespread knowledge of these technological developments mean for user-generated evidence in accountability processes? On the one hand, an overabundance of caution could lead to the exclusion of, or a failure to draw inference from, important and relevant information. On the other hand, inadvertently relying on a deepfake to prove a fact could clearly have devastating effects on the legitimacy and effectiveness of those investigations or trials. In this context, the trust we place in audiovisual content not only has significant consequences in court and on our perceptions of fairness, but can also greatly impact how we document international crimes.
The Burden of Witnessing and Proving Authenticity
Since 2018, WITNESS has been leading a global effort to understand how deepfakes, synthetic media, and generative AI impact democracy, satirical expression, international justice, and human rights advocacy. For over a year, we have also been piloting a taskforce that connects fact-checkers, journalists, and researchers with world-leading media forensics and AI synthesis experts. They provide their knowledge and computational models pro bono, so we can swiftly help those fighting misinformation on the digital frontlines. As part of this initiative, we have received suspected audio fakes from countries such as Sudan, Nigeria, Venezuela, India, and Mexico, as well as other types of potential AI-manipulated content from regions experiencing ongoing conflict or widespread violence.
Our findings indicate that an information ecosystem flooded with synthetic media and more realistic and personalized content (meaning not just personalized feeds, but deceptive information that credibly impersonates non-public figures, sometimes circulated out of public scrutiny) is making it harder to discern truth from falsehood. Increasingly, fact-checkers, journalists, and human rights organizations dealing with audiovisual content, particularly open source information, may question their capacity to verify whether a piece of content has been manipulated – and as WITNESS research has shown, publicly available detection tools have significant limitations.
More generally, this may lead to what Aviv Ovadya has termed “reality apathy”: faced with an increasing stream of misinformation, people simply give up trying to distinguish what is real from what is false. Consultations carried out by WITNESS with grassroots organizations in Latin-America, Africa, the USA, and South-East Asia found that this erosion of trust in the information ecosystem impacts, by extension, the work that civil society organizations (CSOs) are conducting on the ground documenting human rights abuses and international crimes.
When the authenticity of their videos and images can be easily questioned or dismissed, the credibility of CSOs’ damning footage is at risk. This makes it more challenging for these organizations to advocate for justice and accountability. If those documenting human rights are obliged to painstakingly demonstrate the veracity of every piece of content they capture, while those implicated can seamlessly invoke claims of fakery, this risks creating a new form of “epistemic injustice,” where those with the least power are often the least believed.
Two dynamics are particularly troubling in this context. The first is “plausible deniability”. As far back as 2018, Danielle Citron and Robert Chesney wrote of the danger of the “liar’s dividend”. They theorized that, as the public becomes more aware of how AI can be used to generate or manipulate images, audio, and video, then it may become easier for those implicated in real footage to denounce that content as a “deepfake”. Even if it is genuine, the mere possibility that content might have been fabricated casts doubt and may lead to a lack of accountability for real actions. For example, a government accused of committing human rights abuses might claim that the video evidence against them is AI-manipulated, avoiding repercussions.
The second dynamic is “plausible believability”, where realistic-looking manipulated media provides a way for supporters of a cause to cling to their existing beliefs and perpetuate entrenched narratives. For many years, research has documented that people show increased skepticism when presented with material they do not want to see, compared with information that aligns to their preferences. If a piece of user-generated content matches someone’s pre-existing worldview, they are more likely to accept it as true, regardless of its authenticity. This selective acceptance can exacerbate trends of polarization, and makes it more difficult to achieve consensus on basic facts.
As a result, the emergence of deepfakes and synthetic media has introduced a new layer of complexity, where the authenticity of evidence is under heightened scrutiny. Already overburdened journalists, activists, and human rights documenters now face the added challenge of proving that their genuine content is not the product of a sophisticated fabrication. This requires additional resources for verification and corroboration, which can be time-consuming, risky, costly, or out of reach. Missteps can have profound reputational impacts for CSOs, but more pressingly, the increased effort needed to establish credibility can divert resources away from other crucial activities, weakening the overall impact of their work.
Trust in User-Generated Evidence in an AI Era
It is important to establish the landscape of trust in user-generated evidence, in order to examine the impact of both “plausible deniability” and “plausible believability” in practice. The TRUE project seeks to empirically test the hypothesis that the biggest danger with the advent of deepfakes and synthetic media is probably not that fake footage will be introduced in the courtroom, but that real footage will come to be easily dismissed as possibly fake. It has three interlinked investigative clusters:
Legal analysis: mapping how narratives of deepfakes have appeared in cases around the world, and conducting interviews with legal professionals;
Psychological experiments: examining under what conditions user-generated evidence is more or less likely to be believed, and the features of both the evidence and the person viewing it that feed into that assessment;
Mock jury simulations: examining how lay decision-makers reason with and base findings on user-generated evidence in a realistic simulation, and what terms they use to indicate the perceived trustworthiness of that evidence.
Our legal analysis thus far has found that invocations of the so-called “deepfake defense” are perhaps not as common as one might expect, based on in-depth reviews of case law from both international and domestic courts. We have found that user-generated evidence is challenged in a myriad of ways, but it is relatively rare for opponents to imply that it may have been AI-generated.
One notable exception is the case of Ukraine and The Netherlands v. Russia before the European Court of Human Rights. One of the pieces of evidence in this case was a photograph of a Russian soldier named Stanislav Tarasov, which had been posted to his social media profile. This photograph had been used to geolocate him and his military unit to Pavlovka, a site of artillery attacks from Russia to Ukraine in 2014. In fact, there were two slightly different versions of the picture in existence (below); one had a tank number in the background and one did not.
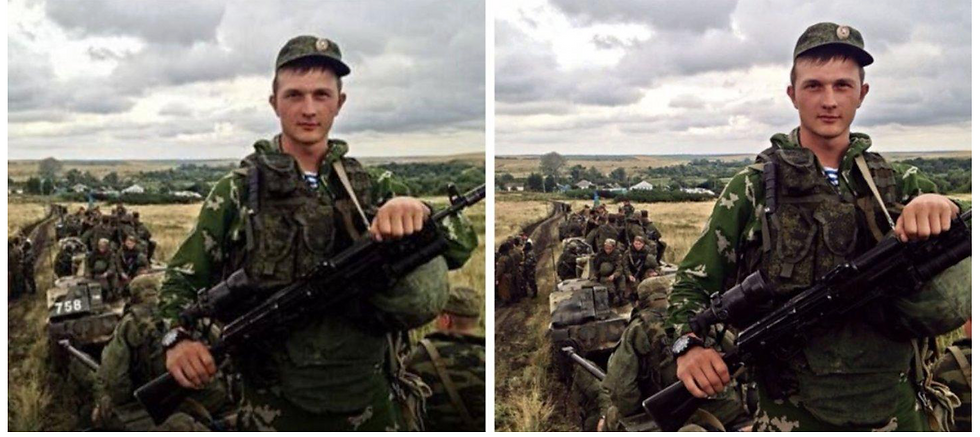
IMAGE: Original (left) and edited (right) photograph of Russian soldier Stanislav Tarasov, originally posted to his social media profile on VK.com.
Before the European Court of Human Rights, Russia seized on this discrepancy to allege that the evidence had been manipulated, focusing on this and what it called other “indications of potential fakery,” including a slight halo around Mr Tarasov, which, it was claimed, “may indicate montage.” Russia added that, “it may be that the entire column [of tanks behind him in the picture] is cloned from a single vehicle.”
Yet, it was later revealed that the soldier had updated his own profile picture on the social media site VK.com to replace the original, which included the tank number, to a version that did not. In its decision of November 2022, the Grand Chamber noted this explanation, adding, “As for the remainder of the photograph, the respondent Government referred only to indications of ‘potential’ fakery. This falls quite significantly short of establishing that the photograph has been fabricated.” This example shows that, perhaps contrary to concerns about the “liar’s dividend” in the courtroom, invoking allegations of manipulation without evidence may not be the most effective legal strategy in seeking to undermine user-generated evidence, even in an era of deepfakes. We saw a similar example in one of the January 6th Capitol insurrection trials, where defense counsel for Guy Reffitt alleged that incriminating evidence might have been a deepfake; Reffitt was nevertheless convicted by a jury in March 2022.
Preparing for a Hybrid AI-Human Media Ecosystem
Even if allegations of AI manipulation have not (yet) deeply impacted legal proceedings, documenters, investigators, legal professionals, and judges will need to consider the challenges posed by the proliferation of synthetic media if we want to safeguard the credibility of user-generated evidence. This section presents key recommendations to ensure that the integrity of audiovisual content is maintained in the face of evolving technology.
Invest in provenance standards and infrastructure: Provenance and authenticity technologies can tell us where and when a piece of footage was taken while maintaining the privacy and security of human rights defenders and documentation teams. Civil society was a pioneer in this area, with tools like eyeWitness, that embeds metadata and a cryptographic hash into photos and videos at the time of capture, being the first and only one with proof of concept in criminal trials. However, the effectiveness of these technologies depends on their widespread adoption and the training of those using them, and there are still many misconceptions concerning the design, development and adoption of provenance technology.
Push for transparency in synthetic media production: With the lines between human and synthetic blurring, it will be hard to address AI content in isolation from the broader question of media provenance. Technical solutions such as watermarks, digital fingerprints, and metadata should be acknowledged in policy, legislative and technical responses, with the necessary nuances to preserve the privacy and anonymity of communities at risk. “Content credentials” – sometimes likened to nutrition labels on food – are increasingly being addedby both hardware and software providers to show the origins of a piece of content, including when AI may have been used.
Develop and deploy detection tools: As the circulation volume of synthetic and hybrid media increases, tools that can detect whether a piece of audiovisual content has been AI-generated or manipulated will become increasingly important for triaging content. Making computational detection work for human rights and conflict contexts will require cooperation and partnerships between the private sector, governments, and CSOs to enhance their effectiveness and mitigate the risks associated with the collection of the data needed to fine-tune them. Although detection tools are still in their infancy and often lag behindgenerative capabilities, investing in their development and making them accessible to those on the frontlines is essential. The need has driven the pilot of WITNESS’s Deepfake Rapid Response Force, which support journalists, fact-checkers and human rights defenders globally by linking them to critical forensic expertise.
Build societal resilience: Technical solutions should be accompanied by other responses that prioritize access to the knowledge and literacy needed to interpret confusing signals of truth. This includes understanding the technology behind deepfakes and the potential for content to be generated or manipulated using AI, as well as the limitations of this technology. These initiatives can help prepare journalists, researchers and open source investigators to examine audiovisual media without adding to the rhetoric around generative AI. Moreover, media literacy can also act as a vehicle for empowering human rights defenders, activists and other key civil society stakeholders to engage with governments and tech companies to develop responses and solutions that reflect societal needs, circumstances and aspirations for justice and accountability for international crimes.
Strengthen legal responses: While some have argued that rules of evidence need to be amended to specifically address the challenges posed by AI, others have noted that raising the bar for admissibility of evidence may have adverse consequences. A helpful middle ground could see judges set out guidelines for the type of information which should accompany audio-visual evidence to assist in its evaluation. This could include information on the metadata; how and where the content was discovered, and its chain of custody, among other relevant indicators. More generally, judges and lawyers need to be educated on the complexities of media manipulation to make informed decisions about the admissibility and weight of digital evidence. This is why we recently co-authored, with other practitioners and academic experts, a guide for judges and fact-finders to assist them in evaluating online digital imagery. We hope the guide, which is available in five languages, will help legal professionals navigate the choppy waters of accountability for human rights violations in an era where trust in evidence is being challenged by developments in AI.
***
User-generated content can provide invaluable evidence of atrocity crimes, but synthetic media is reshaping the landscape of information and trust. Generative AI empowers those who wish to deny or dismiss real events, and creates a protective layer for misinformation. Now, organizations and individuals recording on camera human rights abuses and international crimes face the double challenge of capturing truthful content while overcoming increasingly rising skepticism. This evolving dynamic threatens not only the efforts to document human rights violations and international crimes, but also our ability as a society to deliver justice and ensure accountability.
Comments